Like A/B Testing, Multivariate Testing is based on the same
mechanism, but it compares higher number of variables, and provides more
information about how these variables behave. In A/B Testing, you split
the traffic of a page between different versions of the design.
Multivariate Testing is used to measure the effectiveness of each
design.
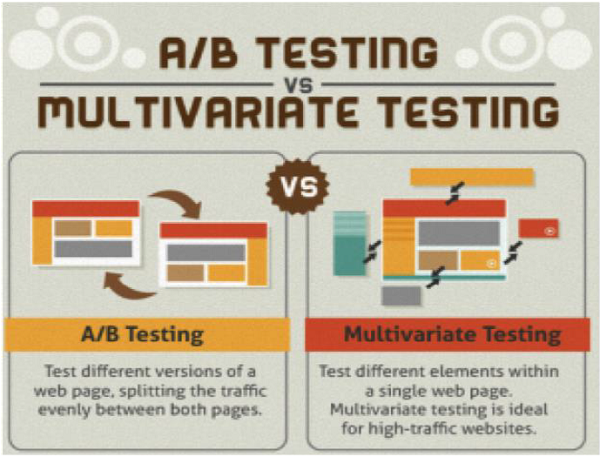
Example
Let us say there is a webpage that has received enough traffic to run the test. Now the data from each variation is compared to check the most successful variation, but it also includes the elements, which have the maximum positive or negative impact on a visitor's interaction.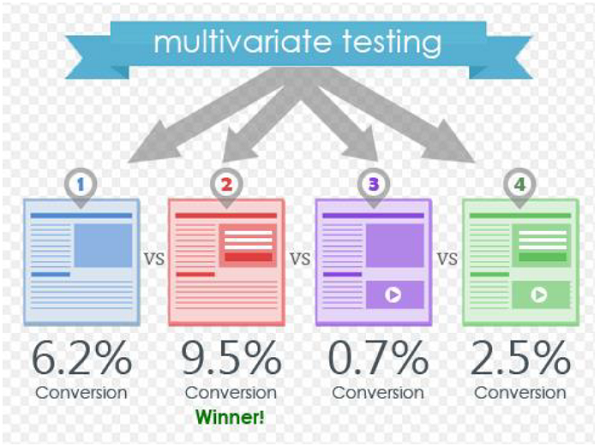
Advantages of using Multivariate
Multivariate Testing is an effective tool to help you target as well as redesign the elements of your page and show the areas that will have the most impact. Multivariate method is useful for creating landing page campaigns.Example
Data about the impact of a certain element's design can be applied to future campaigns, even if the context of the element has changed.Limitations
Limitations of Multivariate testing is the traffic needed to complete the test. As all the experiments are fully factorial, too many changing elements at once can quickly add up to a very large number of possible combinations that must be tested. Even a site with fairly high traffic might have trouble completing a test with more than 25 combinations in a feasible amount of time.Difference between Multivariate and A/B Testing
A/B Testing also known as Split Testing is a method of website optimization, where you compare the conversion rates of two versions of a page namely, A and B. All visitors are divided into one version or the other. Once the visitors visit either of these versions (A or B), they click on various buttons or even sign-up for the newsletter. This allows you to determine which version of the page is more effective.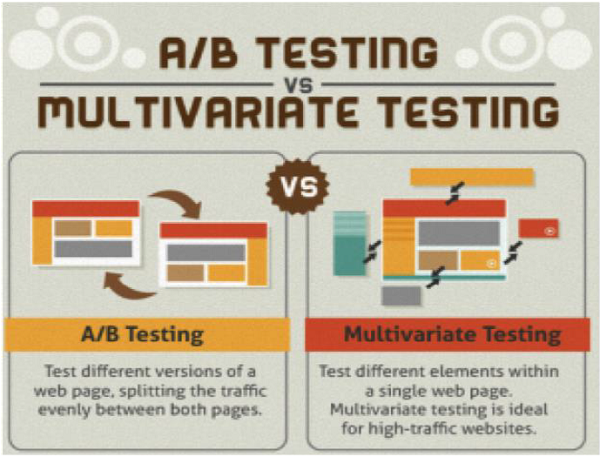
No comments:
Post a Comment